Yinfang CHEN
Ph.D. Candidate
yinfang3 AT illinois DOT edu
Siebel School of Computing and Data Science
The Grainger College of Engineering
University of Illinois at Urbana-Champaign (UIUC)
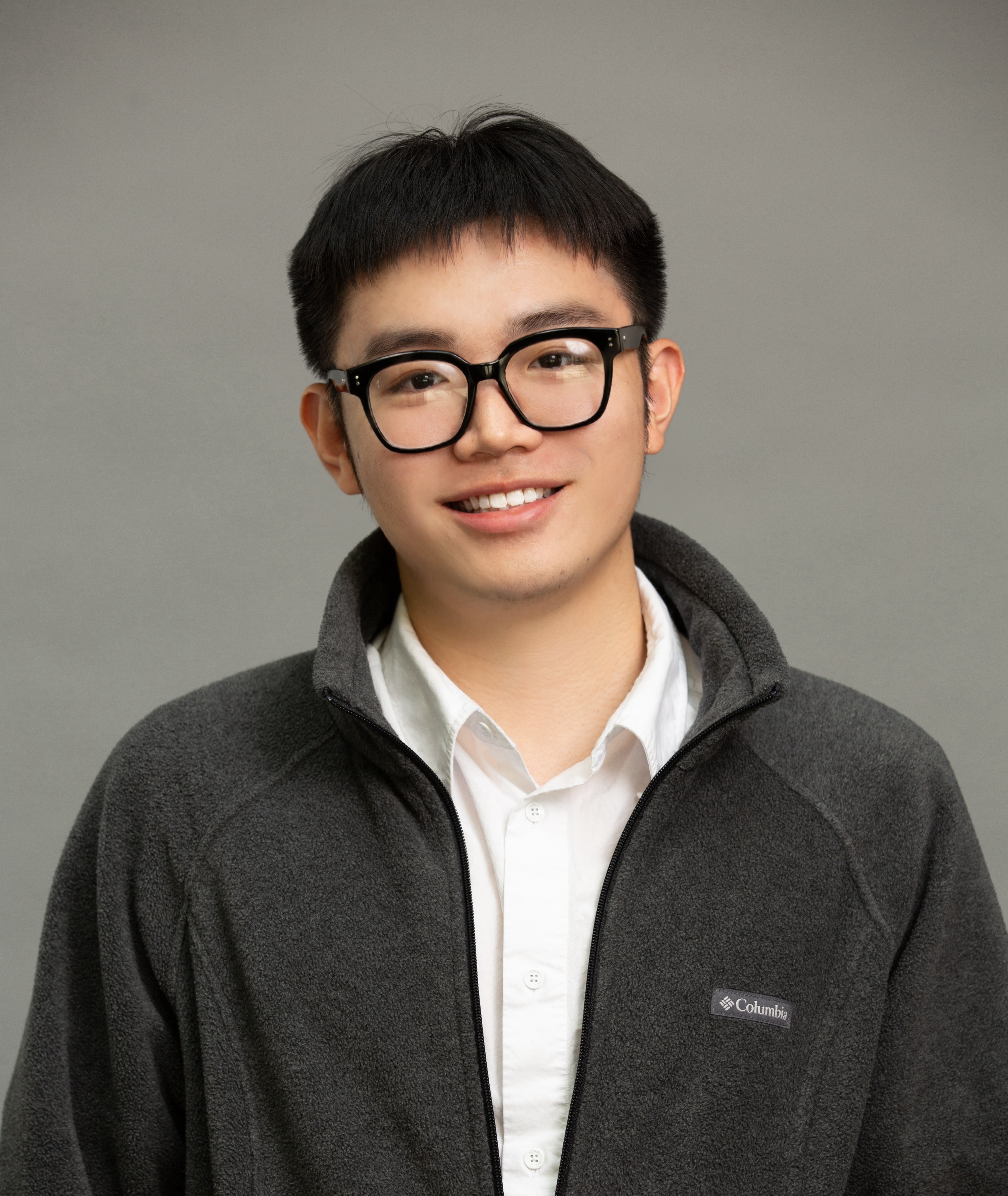
Hi! I am Yinfang Chen, a fourth-year Computer Science Ph.D. candidate “watched” by my “cornfield-watchman-professor” Tianyin Xu in the Department of Computer Science at the University of Illinois at Urbana-Champaign. I earned my Master of Computing degree from the School of Computing (SoC) of the National University of Singapore (NUS). I received my Bachelor’s degree in Computer Science at the Huazhong University of Science and Technology (HUST).
Research Interests
System Reliability, ML for System, System Security, Cloud Computing
News
Feb 11, 2025 | Our work AIOpsLab has been accepted by MLSys’25. Congratulations to the team! |
---|---|
May 13, 2024 | I have joined Microsoft Research as a summer intern! |
Sep 11, 2023 | Our work has been accepted by EuroSys’24!! |
Aug 12, 2023 | I am finally back to the cornfields after ~8 months! |
Aug 1, 2023 | I had GREAT experiences in MSRA and Jialin’s group of NUS! Thanks everyone I met there! |
Feb 1, 2023 | Currently, I am on leave due to an visa issue:( and expect to get back to UIUC in 2023 Fall.. |
Dec 24, 2022 | Our work Rainmaker has been accepted by NSDI’23! Thanks everyone in the project group! |
Selected Papers
- AIOpsLab: A Holistic Framework to Evaluate AI Agents for Enabling Autonomous CloudsYinfang Chen, Manish Shetty, Gagan Somashekar, Minghua Ma, Yogesh Simmhan, Jonathan Mace, Chetan Bansal, Rujia Wang, and Saravan RajmohanMay 2025
AI for IT Operations (AIOps) aims to automate complex operational tasks, such as fault localization and root cause analysis, to reduce human workload and minimize customer impact. While traditional DevOps tools and AIOps algorithms often focus on addressing isolated operational tasks, recent advances in Large Language Models (LLMs) and AI agents are revolutionizing AIOps by enabling end-to-end and multitask automation. This paper envisions a future where AI agents autonomously manage operational tasks throughout the entire incident lifecycle, leading to self-healing cloud systems, a paradigm we term AgentOps. Realizing this vision requires a comprehensive framework to guide the design, development, and evaluation of these agents. To this end, we present AIOPSLAB, a framework that not only deploys microservice cloud environments, injects faults, generates workloads, and exports telemetry data but also orchestrates these components and provides interfaces for interacting with and evaluating agents. We discuss the key requirements for such a holistic framework and demonstrate how AIOPSLAB can facilitate the evaluation of next-generation AIOps agents. Through evaluations of state-of-the-art LLM agents within the benchmark created by AIOPSLAB, we provide insights into their capabilities and limitations in handling complex operational tasks in cloud environments.
@misc{chen2024aiopslab, title = {AIOpsLab: A Holistic Framework to Evaluate AI Agents for Enabling Autonomous Clouds}, author = {Chen, Yinfang and Shetty, Manish and Somashekar, Gagan and Ma, Minghua and Simmhan, Yogesh and Mace, Jonathan and Bansal, Chetan and Wang, Rujia and Rajmohan, Saravan}, year = {2025}, booktitle = {The Eighth Annual Conference on Machine Learning and Systems (MLSys'25)}, month = may, github = {https://github.com/microsoft/AIOpsLab}, url = {https://www.microsoft.com/en-us/research/publication/aiopslab-a-holistic-framework-for-evaluating-ai-agents-for-enabling-autonomous-cloud/}, }
- ITBench: Evaluating AI Agents across Diverse Real-World IT Automation TasksSaurabh Jha, Rohan Arora, Yuji Watanabe, Takumi Yanagawa, Yinfang Chen, Jackson Clark, Bhavya Bhavya, Mudit Verma, Harshit Kumar, Hirokuni Kitahara, Noah Zheutlin, Saki Takano, Divya Pathak, Felix George, Xinbo Wu, Bekir O. Turkkan, Gerard Vanloo, Michael Nidd, Ting Dai, Oishik Chatterjee, and 23 more authorsFeb 2025Featured by IBM Research Blog
Realizing the vision of using AI agents to automate critical IT tasks depends on the ability to measure and understand effectiveness of proposed solutions. We introduce ITBench, a framework that offers a systematic methodology for benchmarking AI agents to address real-world IT automation tasks. Our initial release targets three key areas: Site Reliability Engineering (SRE), Compliance and Security Operations (CISO), and Financial Operations (FinOps). The design enables AI researchers to understand the challenges and opportunities of AI agents for IT automation with push-button workflows and interpretable metrics. ITBench includes an initial set of 94 realworld scenarios, which can be easily extended by community contributions. Our results show that agents powered by state-of-the-art models resolve only 13.8% of SRE scenarios, 25.2% of CISO scenarios, and 0% of FinOps scenarios. We expect ITBench to be a key enabler of AI-driven IT automation that is correct, safe, and fast.
@misc{jha2025itbenchevaluatingaiagents, title = {ITBench: Evaluating AI Agents across Diverse Real-World IT Automation Tasks}, github = {https://github.com/IBM/itbench-sample-scenarios}, author = {Jha, Saurabh and Arora, Rohan and Watanabe, Yuji and Yanagawa, Takumi and Chen, Yinfang and Clark, Jackson and Bhavya, Bhavya and Verma, Mudit and Kumar, Harshit and Kitahara, Hirokuni and Zheutlin, Noah and Takano, Saki and Pathak, Divya and George, Felix and Wu, Xinbo and Turkkan, Bekir O. and Vanloo, Gerard and Nidd, Michael and Dai, Ting and Chatterjee, Oishik and Gupta, Pranjal and Samanta, Suranjana and Aggarwal, Pooja and Lee, Rong and Murali, Pavankumar and Ahn, Jae-wook and Kar, Debanjana and Rahane, Ameet and Fonseca, Carlos and Paradkar, Amit and Deng, Yu and Moogi, Pratibha and Mohapatra, Prateeti and Abe, Naoki and Narayanaswami, Chandrasekhar and Xu, Tianyin and Varshney, Lav R. and Mahindru, Ruchi and Sailer, Anca and Shwartz, Laura and Sow, Daby and Fuller, Nicholas C. M. and Puri, Ruchir}, year = {2025}, month = feb, eprint = {2502.05352}, archiveprefix = {arXiv}, primaryclass = {cs.AI}, }
- Large Language Models as Configuration ValidatorsIn Proceedings of 47th International Conference on Software Engineering (ICSE’25), Apr 2025
Misconfigurations are major causes of software failures. Existing practices rely on developer-written rules or test cases to validate configuration values, which are expensive. Machine learning (ML) for configuration validation is considered a promising direction, but has been facing challenges such as the need of large-scale field data and system-specific models. Recent advances in Large Language Models (LLMs) show promise in addressing some of the long-lasting limitations of ML-based configuration validation. We present the first analysis on the feasibility and effectiveness of using LLMs for configuration validation. We empirically evaluate LLMs as configuration validators by developing a generic LLM-based configuration validation framework, named Ciri. Ciri employs effective prompt engineering with few-shot learning based on both valid configuration and misconfiguration data. Ciri checks outputs from LLMs when producing results, addressing hallucination and nondeterminism of LLMs. We evaluate Ciri’s validation effectiveness on eight popular LLMs using configuration data of ten widely deployed open-source systems. Our analysis (1) confirms the potential of using LLMs for configuration validation, (2) explores design space of LLMbased validators like Ciri, and (3) reveals open challenges such as ineffectiveness in detecting certain types of misconfigurations and biases towards popular configuration parameters.
@inproceedings{lian2025configuration, title = {Large Language Models as Configuration Validators}, author = {Lian*, Xinyu and Chen*, Yinfang and Cheng, Runxiang and Huang, Jie and Thakkar, Parth and Xu, Tianyin}, year = {2025}, month = apr, github = {https://github.com/xlab-uiuc/ciri}, booktitle = {Proceedings of 47th International Conference on Software Engineering (ICSE'25)}, }
- Fidelity of Cloud Emulators: The Imitation Game of Testing Cloud-based SoftwareIn Proceedings of 47th International Conference on Software Engineering (ICSE’25), Apr 2025
Modern software projects have been increasingly using cloud services as important components. The cloud-based programming practice greatly simplifies software development by harvesting cloud benefits (e.g., high availability and elasticity). However, it imposes new challenges for software testing and analysis, due to opaqueness of cloud backends and monetary cost of invoking cloud services for continuous integration and deployment. As a result, cloud emulators are developed for offline development and testing, before online testing and deployment. This paper presents a systematic analysis of cloud emulators from the perspective of cloud-based software testing. Our goal is to (1) understand the discrepancies introduced by cloud emulation with regard to software quality assurance and deployment safety and (2) address inevitable gaps between emulated and real cloud services. The analysis results are concerning. Among 255 APIs of five cloud services from Azure and Amazon Web Services (AWS), we detected discrepant behavior between the emulated and real services in 94 (37%) of the APIs. These discrepancies lead to inconsistent testing results, threatening deployment safety, introducing false alarms, and creating debuggability issues. The root causes are diverse, including accidental implementation defects and essential emulation challenges. We discuss potential solutions and develop a practical mitigation technique to address discrepancies of cloud emulators for software testing.
@inproceedings{mazhar2025fidelity, title = {Fidelity of Cloud Emulators: The Imitation Game of Testing Cloud-based Software}, author = {Mazhar, Anna and Alam, Saad Sher and Zheng, William and Chen, Yinfang and Nath, Suman and Xu, Tianyin}, year = {2025}, month = apr, booktitle = {Proceedings of 47th International Conference on Software Engineering (ICSE'25)}, }
- Automatic Root Cause Analysis via Large Language Models for Cloud IncidentsYinfang Chen, Huaibing Xie, Minghua Ma, Yu Kang, Xin Gao, Liu Shi, Yunjie Cao, Xuedong Gao, Hao Fan, Ming Wen, Jun Zeng, Supriyo Ghosh, Xuchao Zhang, Chaoyun Zhang, Qingwei Lin, Saravan Rajmohan, Dongmei Zhang, and Tianyin XuIn Proceedings of the 19th European Conference on Computer Systems (EuroSys’24), Apr 2024Deployed at Microsoft
Ensuring the reliability and availability of cloud services necessitates efficient root cause analysis (RCA) for cloud incidents. Traditional RCA methods, which rely on manual investigations of data sources such as logs and traces, are often laborious, error-prone, and challenging for on-call engineers. In this paper, we introduce RCACopilot, an innovative on-call system empowered by the large language model for automating RCA of cloud incidents. RCACopilot matches incoming incidents to corresponding incident handlers based on their alert types, aggregates the critical runtime diagnostic information, predicts the incident’s root cause category, and provides an explanatory narrative. We evaluate RCACopilot using a real-world dataset consisting of a year’s worth of incidents from Microsoft. Our evaluation demonstrates that RCACopilot achieves RCA accuracy up to 0.766. Furthermore, the diagnostic information collection component of RCACopilot has been successfully in use at Microsoft for over four years.
@inproceedings{chen2023automatic, title = {Automatic Root Cause Analysis via Large Language Models for Cloud Incidents}, author = {Chen, Yinfang and Xie, Huaibing and Ma, Minghua and Kang, Yu and Gao, Xin and Shi, Liu and Cao, Yunjie and Gao, Xuedong and Fan, Hao and Wen, Ming and Zeng, Jun and Ghosh, Supriyo and Zhang, Xuchao and Zhang, Chaoyun and Lin, Qingwei and Rajmohan, Saravan and Zhang, Dongmei and Xu, Tianyin}, booktitle = {Proceedings of the 19th European Conference on Computer Systems (EuroSys'24)}, year = {2024}, month = apr, }
- Building AI Agents for Autonomous Clouds: Challenges and Design PrinciplesManish Shetty, Yinfang Chen, Gagan Somashekar, Minghua Ma, Yogesh Simmhan, Xuchao Zhang, Jonathan Mace, Dax Vandevoorde, Pedro Las-Casas, Shachee Mishra Gupta, Suman Nath, Chetan Bansal, and Saravan RajmohanIn Proceedings of 15th ACM Symposium on Cloud Computing (SoCC’24), Nov 2024Featured by Microsoft Research Blog
The rapid growth in the use of Large Language Models (LLMs) and AI Agents as part of software development and deployment is revolutionizing the information technology landscape. While code generation receives significant attention, a higher-impact application lies in using AI agents for operational resilience of cloud services, which currently require significant human effort and domain knowledge. There is a growing interest in AI for IT Operations(AIOps) which aims to automate complex operational tasks, like fault localization and root cause analysis, thereby reducing human intervention and customer impact. However, achieving the vision of autonomous and self-healing clouds though AIOps is hampered by the lack of standardized frameworks for building, evaluating, and improving AIOps agents. This vision paper lays the groundwork for such a framework by first framing the requirements and then discussing design decisions that satisfy them. We also propose AIOpsLab, a prototype implementation leveraging agent-cloudinterface that orchestrates an application, injects real-time faults using chaos engineering, and interfaces with an agent to localize and resolve the faults. We report promising results and lay the groundwork to build a modular and robust framework for building,evaluating, and improving agents for autonomous clouds.
@inproceedings{shetty2024building, title = {Building AI Agents for Autonomous Clouds: Challenges and Design Principles}, author = {Shetty, Manish and Chen, Yinfang and Somashekar, Gagan and Ma, Minghua and Simmhan, Yogesh and Zhang, Xuchao and Mace, Jonathan and Vandevoorde, Dax and Las-Casas, Pedro and Gupta, Shachee Mishra and Nath, Suman and Bansal, Chetan and Rajmohan, Saravan}, year = {2024}, booktitle = {Proceedings of 15th ACM Symposium on Cloud Computing (SoCC'24)}, month = nov, }
- Push-Button Reliability Testing for Cloud-Backed Applications with RainmakerIn Proceedings of the 20th USENIX Symposium on Networked Systems Design and Implementation (NSDI’23), Apr 2023Featured by The Weekend Read
Modern applications have been emerging towards a cloudbased programming model where applications depend on cloud services for various functionalities. Such “cloud native” practice greatly simplifies application deployment and realizes cloud benefits (e.g., availability). Meanwhile, it imposes emerging reliability challenges for addressing fault models of the opaque cloud and less predictable Internet connections. In this paper, we discuss these reliability challenges. We develop a taxonomy of bugs that render cloud-backed applications vulnerable to common transient faults. We show that (mis)handling transient error(s) of even one REST call interaction can adversely affect application correctness. We take a first step to address the challenges by building a “push-button” reliability testing tool named Rainmaker, as a basic SDK utility for any cloud-backed application. Rainmaker helps developers anticipate the myriad of errors under the cloud-based fault model, without a need to write new policies, oracles, or test cases. Rainmaker directly works with existing test suites and is a plug-and-play tool for existing test environments. Rainmaker injects faults in the interactions between the application and cloud services. It does so at the REST layer, and thus is transparent to applications under test. More importantly, it encodes automatic fault injection policies to cover the various taxonomized bug patterns, and automatic oracles that embrace existing in-house software tests. To date, Rainmaker has detected 73 bugs (55 confirmed and 51 fixed) in 11 popular cloud-backed applications.
@inproceedings{chen2023push-button, title = {Push-Button Reliability Testing for Cloud-Backed Applications with Rainmaker}, author = {Chen, Yinfang and Sun, Xudong and Nath, Suman and Yang, Ze and Xu, Tianyin}, booktitle = {Proceedings of the 20th USENIX Symposium on Networked Systems Design and Implementation (NSDI'23)}, year = {2023}, month = apr, url = {https://www.microsoft.com/en-us/research/publication/push-button-reliability-testing-for-cloud-backed-applications-with-rainmaker/}, github = {https://github.com/xlab-uiuc/rainmaker}, }
- SoK: History is a Vast Early Warning System: Auditing the Provenance of System IntrusionsMuhammad Adil Inam, Yinfang Chen, Akul Goyal, Jason Liu, Jaron Mink, Noor Michael, Sneha Gaur, Adam Bates, and Wajih Ul HassanIn Proceedings of the 44th IEEE Symposium on Security and Privacy (S&P’23), May 2023
Auditing, a central pillar of operating system security, has only recently come into its own as an active area of public research. This resurgent interest is due in large part to the notion of data provenance, a technique that iteratively parses audit log entries into a dependency graph that explains the history of system execution. Provenance facilitates precise threat detection and investigation through causal analysis of sophisticated intrusion behaviors. However, the absence of a foundational audit literature, combined with the rapid publication of recent findings, makes it difficult to gain a holistic picture of advancements and open challenges in the area. In this work, we survey and categorize the provenance-based system auditing literature, distilling contributions into a layered taxonomy based on the audit log capture and analysis pipeline. Recognizing that the Reduction Layer remains a key obstacle to the further proliferation of causal analysis technologies, we delve further on this issue by conducting an ambitious independent evaluation of 8 exemplar reduction techniques against the recently-released DARPA Transparent Computing datasets. Our experiments uncover that past approaches frequently prune an overlapping set of activities from audit logs, reducing the synergistic benefits from applying them in tandem; further, we observe an inverse relation between storage efficiency and anomaly detection performance. However, we also observe that log reduction techniques are able to synergize effectively with data compression, potentially reducing log retention costs by multiple orders of magnitude. We conclude by discussing promising future directions for the field.
@inproceedings{inam2023sok, title = {SoK: History is a Vast Early Warning System: Auditing the Provenance of System Intrusions}, author = {Inam, Muhammad Adil and Chen, Yinfang and Goyal, Akul and Liu, Jason and Mink, Jaron and Michael, Noor and Gaur, Sneha and Bates, Adam and Hassan, Wajih Ul}, booktitle = {Proceedings of the 44th IEEE Symposium on Security and Privacy (S&P'23)}, pages = {307--325}, year = {2023}, month = may, organization = {IEEE}, }
- Shadewatcher: Recommendation-guided cyber threat analysis using system audit recordsIn Proceedings of the 43rd IEEE Symposium on Security and Privacy (S&P’22), May 2022
System auditing provides a low-level view into cyber threats by monitoring system entity interactions. In response to advanced cyber-attacks, one prevalent solution is to apply data provenance analysis on audit records to search for anomalies (anomalous behaviors) or specifications of known attacks. However, existing approaches suffer from several limitations: 1) generating high volumes of false alarms, 2) relying on expert knowledge, or 3) producing coarse-grained detection signals. In this paper, we recognize the structural similarity between threat detection in cybersecurity and recommendation in information retrieval. By mapping security concepts of system entity interactions to recommendation concepts of user-item interactions, we identify cyber threats by predicting the preferences of a system entity on its interactive entities. Furthermore, inspired by the recent advances in modeling high-order connectivity via item side information in the recommendation, we transfer the insight to cyber threat analysis and customize an automated detection system, SHADEWATCHER. It fulfills the potential of high-order information in audit records via graph neural networks to improve detection effectiveness. Besides, we equip SHADEWATCHER with dynamic updates towards better generalization to false alarms. In our evaluation against both real-life and simulated cyber-attack scenarios, SHADEWATCHER shows its advantage in identifying threats with high precision and recall rates. Moreover, SHADEWATCHER is capable of pinpointing threats from nearly a million system entity interactions within seconds.
@inproceedings{zeng2022shadewatcher, title = {Shadewatcher: Recommendation-guided cyber threat analysis using system audit records}, author = {Zeng, Jun and Wang, Xiang and Liu, Jiahao and Chen, Yinfang and Liang, Zhenkai and Chua, Tat-Seng and Chua, Zheng Leong}, booktitle = {Proceedings of the 43rd IEEE Symposium on Security and Privacy (S&P'22)}, pages = {489--506}, year = {2022}, month = may, organization = {IEEE}, github = {https://github.com/jun-zeng/ShadeWatcher}, }
- WATSON: Abstracting Behaviors from Audit Logs via Aggregation of Contextual SemanticsIn Proceedings of the 28th Annual Network and Distributed System Security Symposium (NDSS’21), Feb 2021
Endpoint monitoring solutions are widely deployed in today’s enterprise environments to support advanced attack detection and investigation. These monitors continuously record system-level activities as audit logs and provide deep visibility into security incidents. Unfortunately, to recognize behaviors of interest and detect potential threats, cyber analysts face a semantic gap between low-level audit events and high-level system behaviors. To bridge this gap, existing work largely matches streams of audit logs against a knowledge base of rules that describe behaviors. However, specifying such rules heavily relies on expert knowledge. In this paper, we present WATSON, an automated approach to abstracting behaviors by inferring and aggregating the semantics of audit events. WATSON uncovers the semantics of events through their usage context in audit logs. By extracting behaviors as connected system operations, WATSON then combines event semantics as the representation of behaviors. To reduce analysis workload, WATSON further clusters semantically similar behaviors and distinguishes the representatives for analyst investigation. In our evaluation against both benign and malicious behaviors, WATSON exhibits high accuracy for behavior abstraction. Moreover, WATSON can reduce analysis workload by two orders of magnitude for attack investigation.
@inproceedings{zeng2021watson, title = {WATSON: Abstracting Behaviors from Audit Logs via Aggregation of Contextual Semantics}, author = {Zeng, Jun and Chua, Zheng Leong and Chen, Yinfang and Ji, Kaihang and Liang, Zhenkai and Mao, Jian}, booktitle = {Proceedings of the 28th Annual Network and Distributed System Security Symposium (NDSS'21)}, year = {2021}, month = feb, }